Simulation and machine learning for future medicine
We worked with AstraZeneca to accelerate lead optimisation by providing insights on a new class of drugs with the potential to reduce costs and increase productivity.
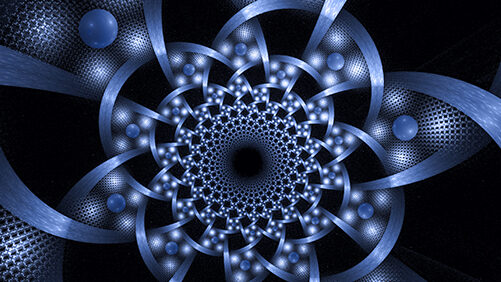
Challenge
The cost of developing new, successful drugs is soaring with huge implications for productivity. To overcome this challenge, the life science sector is investigating new modalities such as peptides and oligonucleotides that could expand the way new drugs can bind to target proteins. Cyclic peptides, small proteins that are looped together, are promising new modalities that can interact with disease targets that have previously been unsuccessfully targeted by small molecules. For a drug to be effective, binding to a target is not enough, it must also be able to cross the cell membrane. The ability to predict and improve membrane permeability of cyclic peptides is a key priority for the AstraZeneca team.
Approach
The team developed computational workflows that blend molecular dynamics, a technique that simulates the movements of atoms and molecules on a supercomputer, with machine learning. They used dimensionality reduction and clustering, both machine learning techniques, to identify patterns of movement and study the shapes of the cyclic peptides with the AstraZeneca team. This provided an insight into how chemical modifications influence shape and therefore permeability. The team also created user-friendly computational notebooks, allowing domain scientists to access supercomputers and provide computational building blocks such as simulation, visualisation and analysis into a single, interactive user interface.
Benefits
This work, completed as part of the Innovation Return on Research (IROR) programme, a collaboration between STFC and IBM Research, can improve lead optimisation by potentially making a drug more permeable or easy to manufacture, saving costs and boosting productivity. This novel approach to making computational notebooks accessible on supercomputers also helps democratise computational science, allowing domain scientists easy access to high performance computational workflows and analytics.
“We are excited to build on this collaboration and work towards large scale benchmarking exercises and adoption of machine learning capabilities. Our collaboration with IBM and the Hartree Centre has resulted in new insights into a challenging system and a demonstration of exciting new tools for drug discovery.”
Anders Hogner, AstraZeneca
Join Newsletter
Provide your details to receive regular updates from the STFC Hartree Centre.