Boulder detection from seabed sonar images
We worked with hydrographic survey company Bibby Hydromap, using computational deep learning techniques to automate and accelerate the process of labelling boulders in sonar images.
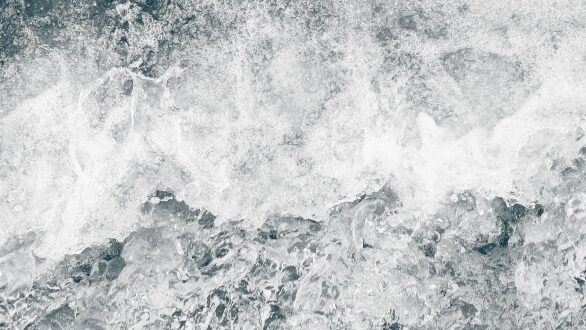
Challenge
Exploring the seabed remains challenging as it is difficult for humans to explore directly, and raw sonar images are usually greyscale and noisy. Currently, identifying boulders on the sea bed from data generated from survey ships is a manual process completed by experienced geophysicists. In order to enable better planning for offshore developments, Bibby Hydromap were looking to find ways to automate the annotation of raw side scan sonar images, reducing the significant time and resources associated with manual boulder labelling.
Approach
The team first prepared the training dataset by dividing one large image (~500MB) – covering a 200sqm seabed area – into 10,000 overlapping smaller tiles. Around 2000 of these tiles were selected randomly for the training dataset, half of them containing multiple boulders labelled by the experts. They went on to use advanced deep learning techniques – capable of learning from large amounts of data – to automate the annotation of raw sonar images. The team then developed and trained a convolutional neural network (CNN), capable of analysing visual images, to perform object detection. The trained CNN rapidly and automatically detected boulders on the seabed in thousands of test images.
Benefits
The trained deep learning model was able to accelerate boulder detection by annotating large raw datasets in less than 30 minutes using a standard desktop computer and in only a few minutes using the IBM POWER8 supercomputer. Developed as part of the Innovation Return on Research (IROR) programme, a collaboration between STFC and IBM Research – the model allows Bibby Hydromap to scale up their business by reducing the time and labour costs associated with identifying boulders on the seabed, and potentially enabling in-situ data processing on survey vessels. This will ultimately help in planning for sub-marine cable placement and offshore renewable energy development.
“This project gave an insight into how machine learning technologies can enhance elements of our business and remove low risk decisions on target identification, leaving our geophysicists to interpret more difficult targets.”
Chris Ferguson, Bibby Hydromap
“Image recognition of targets within side scan sonar using deep learning techniques will improve our productivity while reducing costs, so the final product can be provided to the client within tight time scales.”
Rob White, Bibby Hydromap
Join Newsletter
Provide your details to receive regular updates from the STFC Hartree Centre.